The future of identity is leading conversations across the digital marketing ecosystem. As privacy-first becomes the mantra, how will the industry approach solving for identity? Let’s delve deeper to understand how we got here and what the future will look like from this lens.
Brands have worked tooth and nail to be visible to their audience in every way possible. From massive hoardings & radio messages to TVCs & print ads, marketers used them all- an era in which reach was everything. As customers transitioned to digital platforms, so did brands and their marketing efforts. With it came the realization of how significant accurate targeting is, and that it can be both cost effective and highly relevant. Since then, the approach that data-driven marketing should solve for accurate targeting has inevitably led to identity matching solutions. Chasing this accuracy eventually led to a high dependency on deterministic data.
Deterministic Data Matching
Deterministic data relies on definitive proof of a user’s identity, like first part-data. Identity solutions using deterministic data integrate unique identifiers across multiple touchpoints and look for an exact match across different devices and data sets. The identifiers are primarily personally identifiable information (PII) like email address, phone number, address, date of birth.
Uniquely identifying customers prioritized accuracy and limited the scope of false positives while targeting an audience. Marketers could now marry their first party data with a DMP who would create ID graphs, helping brands recognise patterns at an individual customer level and plan a campaign around it. This worked extremely well when brands wanted to retarget their customers to cross-sell or use a specific line of messaging.
Given the returns on these campaigns, using identity matching became prevalent. The wide usage of deterministic data then brought to attention the limitations that came with it. The two primarily being:
– Privacy of the users, since there wasn’t any consent taken by brands to be able to use customer data to target them elsewhere on the web
– Scalability for marketers seeking new customer acquisition, since they would have to reach new audiences for this and not existing customers
It’s worth mentioning that a number of companies are racing to provide alternatives in a privacy-compliant way. The resulting universal identifiers — from companies including ID5, LiveRamp, Zeotap, and The Trade Desk (UID 2.0), MediaMath— offer an interoperable way of tracking users. Like UID 2.0 uses consumers’ anonymized email addresses which is gathered from a user logging into a website or app (mobile or connected TV). The identifier regularly regenerates itself, ensuring security. SOURCE, the MediaMath approach, looks at shifting from 3P cookies to first-party data completely and creating an end-to-end ecosystem with premium publisher partnerships. SOURCE, similarly, creates a person ID to provide a user match between advertiser’s first party data and the publisher’s site visitors. The advantage of these IDs is that along with identity match – user consent and opt-outs can be managed in a streamlined fashion.
However, Google has said that its Chrome browser will not support solutions like UID 2.0 as they don’t seem like sustainable solutions given the evolving privacy requirements in the industry. But there are several other open questions that solutions like these have left the industry with:
- How do you scale across the supply? especially with mid to small sized publishers who might not get users to share their details easily
- Another walled garden? There’s a risk of this happening in order to reach certain publishers who may be tied to a Universal ID solution
- Getting user’s consent – The solutions will still need users to opt-in to sharing and using their information to show them ads
Probabilistic Data Matching
The looming concerns around individual identity led marketers to try out more cohort-based solutions that use probabilistic data. Probabilistic data matching is usually based on behavioural data, appography, etc that are aggregated and analysed in order to determine the probability that a user belongs to a certain demographic or interest category. From our browsing habits we create distinct behavioural patterns that often can be identified algorithmically in anonymised log files.
Probabilistic data is pulled from these large group of datasets to create a buyer persona. Advanced algorithms segment these audiences by topics they are research, behaviours they exhibit and/or the likelihood of them performing certain actions in the future. It uses anonymised data points to segregate users based on online behaviour. This approach relies on predictive modelling and/or aggregation of users. These are the fundamentals of cohorts-based solutions.
Cohort-based Data Matching
This method focuses on combining groups of people in signed-in universes with common interests into cohorts. Advertisers target these cohorts instead of individual people, making it a very viable alternative for a privacy led ecosystem.
A cohort stands for a group of users that fall under common criteria. For example, cohorts could be:
- “Every user who bought Nike shoes”
- “Every user who made a purchase on the website in January 2021”
- “Every consumer acquired from a Facebook ad”
Let’s very quickly get a sense of the Deterministic vs Cohorts based debate:
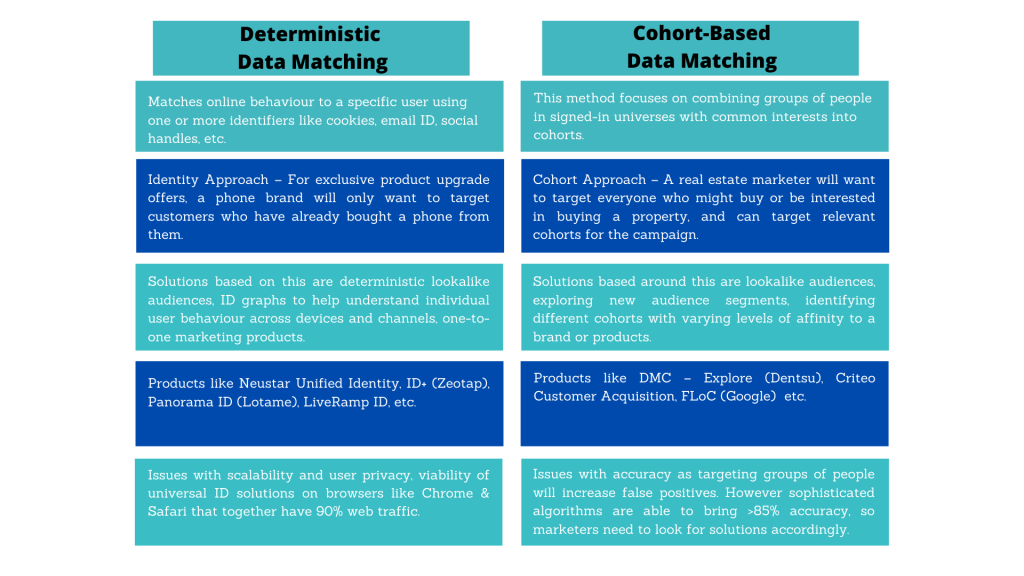
Why is Cohort-based data matching the future?
In light of a cookie-less future, it can be said that some solutions based on deterministic data have their days numbered since many of these still use cookie matching or link IDs to generate individual level audience insights. But with privacy laws now in place, being able to track an individual’s browsing patterns will be a serious violation (unless there’s user consent). As an industry we should aim at protecting consumer data to the best of our ability, this will also go a long way in helping brands build trust with their customers. Hence there is a need to pivot towards an approach that’s secure for the consumers and sustainable for marketers, this is exactly what probabilistic data matching solutions address.
With Dentsu Marketing Cloud, our fundamental focus has always been to replace individual identifiers with groups of people who have common interests. And four years ago, we were able to generate just that – a privacy by design product suite. By using large sets of cohorts-based data, we built an ecosystem to help brands understand and connect with their audience cohorts without an emphasis on one-to-one enrichment, but in many-to-many enhancement and targeting processes. The Dentsu Marketing Cloud proposed a way for brands to connect and engage with their audiences based on clustering audience groups. Audience with relevant interests and behavioural signals derived in a privacy focused manner that encompasses all signed-in data within large ecosystems such as Facebook and Google. We’re able to help marketers determine audiences that most engaged with their brand or product or interest-based classification.
The Data Science team at Dentsu has always taken a firm stance on the deterministic vs probabilistic debate: probabilistic data matching is the present and the future. It makes for a scalable and privacy complaint way to reach your audience, and it doesn’t require a heavy reliance on first part data. Our cohort-based ecosystem enabling 5000+ client campaigns across varied spend levels and advertising objectives, the Dentsu Marketing Cloud has delivered an average efficiency improvement of 25%.
Additionally, it’s been encouraging to see that Big Data companies like Google are also taking a cohort-based route in their path to being a more privacy focused solution. The Federated Learning of Cohorts (FLoC) proposed by Google’s Privacy Sandbox is a cohort-based model that introduces a new way for businesses to reach people with relevant content and ads by clustering large groups of people with similar interests.
Enabling a privacy-first led future gives us the liberty to rethink the digital marketing ecosystem. We must take advantage of this to encourage and build better brand-audience relationships that foster an identity foundation based on user consent.